Editor’s Note: For more on AI in thoracic oncology, read about current and future implications from Raymond H. Mak, MD, and Fridolin Haugg, MSc.
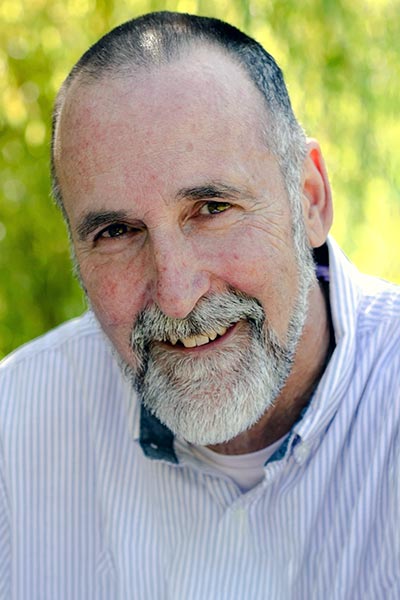
If it feels like discussions of artificial intelligence—and its endless promises and possibilities—are everywhere, there is a good reason for that. AI use and research has grown rapidly in recent years. A Google Scholar search for “lung cancer artificial intelligence” yields nearly 5,000 results in 2023 alone.
The advances are exciting, but as a patient with a life-threatening condition, it often feels excitement overrules caution. As patients, we need to know if these new systems are safe. Another way to frame it: Do I trust AI to make decisions about my health?
AI pioneer IBM defines artificial intelligence as “a field which combines computer science and robust datasets to enable problem-solving.” The possibilities for the use of AI in lung cancer healthcare are myriad. Examples include:
- Identification of high-risk patients for screening;
- Interpretation of low dose CT scans;
- AI-assisted robotic surgery;
- Radiation treatment planning;
- Biomarker analysis;
- Identification of patients in need of palliative intervention;
- Communication coaching for difficult physician-patient conversations
In May, physician scientist Eric Topol discussed an AI tool that has been developed to transcribe a patient-doctor conversation and provide feedback to the doctor about how they could have improved the interaction.
For the patient, AI is not just the latest, greatest technological toy but potentially the thing that could save their lives—or kill them. The potential for this to go horribly wrong is huge. And if we get it wrong, the risk for the patient is death.
Recently, a group of influential tech leaders called for a 6-month moratorium on AI research and development, raising some important questions. In part, the controversial open letter reads “AI research and development should be refocused on making today’s powerful, state-of-the-art systems more accurate, safe, interpretable, transparent, robust, aligned, trustworthy, and loyal.”
As a patient watching the rise of AI, I’ve considered each of these issues, and I will explore them here in the context of lung cancer care. My hope is more stakeholders will pause to consider these issues as well so we ensure the AI systems we adopt can fulfill their promises without compromising patient safety and trust.
Accuracy & Safety
AI systems require data. Ensuring the data is valid and credible is vital to the accuracy and safety of any AI-generated output. Unfortunately, we have already seen AI systems trained on data that reflects biases in ethnographic and cultural distribution.1
Bias can creep in in unexpected ways. UNESCO, in their paper on AI ethical issues, suggests an experiment: compare the search engine results of “Doctor” versus “Nurse.” The images that appear vividly reflect the societal stereotypes and gender bias introduced in algorithms and datasets. These biases can have far-reaching effects. Extrapolate these biases into the realm of lung cancer screening or treatment and the effects could be life-threatening.
Beyond biases, data can be inaccurate for any number of other reasons. A frequently cited promise of AI is its potential to improve the quality of the data collection with better record transcription.
Can AI be used to improve data collection, address inconsistencies, and thus improve the safety and accuracy of AI? Perhaps. Anyone who has spent time looking at patient records knows they frequently include inaccuracies, so the status quo is not without its own problems.
Another of the great promises of AI technology is its potential to save doctors from acting as data clerks. Freeing clinicians from data entry work would allow them to spend more time with their patients, but is AI the answer? Recently, a friend reviewed the notes from a recent medical appointment. The notes were filled with egregious inaccuracies. Would AI have helped prevent these inaccuracies? Or would it have amplified them?
Potentially one of the areas of greatest AI impact will be radiology. Digital tools are already being used for image-improvement, image-quantification, and decision-making. Computer vision is much more sensitive than the human eye for image interpretation, and AI has consistently shown it can see things in scans that radiologists do not see. The field has also struggled with how to leverage imaging data to solve clinical problems, and the advent of AI may help address such challenges.
But is it safe? And how safe is safe? For me, as a consumer, if an airline safely lands 99.9% of 1,000 flights, there would still be one crash. That level of risk would be unacceptable—aviation safety demands 100% accuracy.
As a patient, I want—and we should demand—that type of accuracy from AI as well. AI researchers and proponents could learn a lot about risk management and safety from the airline industry.
Interpretation & Robustness
The software used to work with large datasets is complex and on a certain level does not have clear logic. Rather, the logic is “fuzzy.” Understanding why AI makes certain recommendations—and thus interpreting them—can be difficult.
This leads to what McCradden and Kirsch, a team of researchers from the Hospital for Sick Children in Toronto, have called “AI paternalism.” Rather than questioning the AI recommendation, we accept it. In their words, “…replacing the all-knowing doctor with the all-knowing AI” is another form of medical paternalism.2 This must be addressed if AI is to be a useful tool in healthcare.
And like the accuracy of data behind AI, the robustness of the data must also be considered. More than the strength and vigor usually envisioned, the use of the term robustness in technology implies that AI can handle a wide range of data. Indeed, it must handle the full range, including what researchers often refer to as “edge cases” or “outliers,” because they lie beyond the range of normal data and accounting for them can be difficult.
A statistician takes robust data to mean that the results are resistant to error. For the patient, robust data means that the data accounts for their demographic in the modelling of their lung cancer.
Trust & Transparency—Will the real doctor please stand up?
When medical decisions are being reached, patients need to know if a machine is making the decision. Ranging from a note on a medical record, to an information label on a machine, this transparency can take many forms. Systems incorporating AI need to be visible.
Access to the technology is another concern. Developing AI technology demands large data sets that are expensive. Ensuring that the technology does not become cost-prohibitive and is accessible on a global scale is a challenge. For example, some teams have already run into an unwillingness from data collectors to share their dataset training images to examine them for bias. The secrecy required to maintain a competitive edge may work against the development of trust in these systems.
When AI tools do not have clear data, they can “hallucinate” appropriate data. Indeed, scientific journals have seen false references in abstracts written with the help of AI.3
Alignment & Loyalty
Most AI implementation strategies are using some form of human interaction to confirm results. Ensuring that the decisions reached are in line with the best practices and current standards of care is key. Data (and by inference AI) may not necessarily tell a clinician everything they need to know about how a patient’s treatment should proceed.
Today, doctors and patients reach treatment decisions together, incorporating the patient’s values. Advances in AI use must not diminish the patient role in decision-making. Keeping the best interests of the patient in mind is of foremost importance.
Greed, profit, or other motives can subvert medical decisions, so AI tools must be vetted to ensure they reliably prioritize the well-being of the patient.
Returning to the issue of trust, patients need to have their concerns about AI technology heard and addressed.
One of my friends likes to tell the story of a person crossing Niagara Falls pushing a wheelbarrow on a wire. They offer to take you across in the wheelbarrow. It is one thing to believe that they can do it. It is quite another to trust them enough to climb into the wheelbarrow and let them wheel you across.
As we climb into the wheelbarrow of AI technology, we need to be careful not to cede our humanity and autonomy.
References
- 1. Sourlos N, Wang J, Nagaraj Y, van Ooijen P, Vliegenthart R. Possible Bias in Supervised Deep Learning Algorithms for CT Lung Nodule Detection and Classification. Cancers (Basel). 2022;14(16):3867. Published 2022 Aug 10. doi:10.3390/cancers14163867
- 2. McCradden, M.D., Kirsch, R.E. Patient wisdom should be incorporated into health AI to avoid algorithmic paternalism. Nat Med 29, 765–766 (2023). https://doi.org/10.1038/s41591-023-02224-8
- 3. Else H. Abstracts written by ChatGPT fool scientists. Nature. 2023;613(7944):423. doi:10.1038/d41586-023-00056-7