Editor’s Note: This editorial is the first in a series of articles and commentaries on artificial intelligence and machine learning in the field of thoracic oncology. Look for more in-depth discussions in the coming weeks as we explore how AI will affect the practice of thoracic oncology—from screening and diagnosis to clinical decision making.
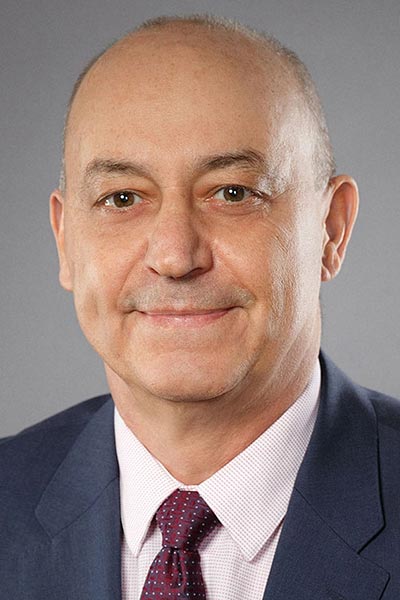
We live in interesting times. On one hand, the intensity of our workload is increasing, especially in fields dealing with broadly understood diagnostics, such as pathology or radiology, as aging populations and electronic medical records lead to more patients in our clinics. On the other hand, we are contending with the demand and availability of new technology—such as robotic solutions in surgical fields—as well as the need for systems that allow for the development of large dataset analyses in pursuit of more precise personalized medicine. By the same token, we are observing mounting development and interest in the use of machine learning and artificial intelligence as a solution to these needs. According to tech intelligence firm CB Insights, AI sector funding increased by 108% in 2021. We can expect AI to enter our clinical practice. The question is, to what extent?
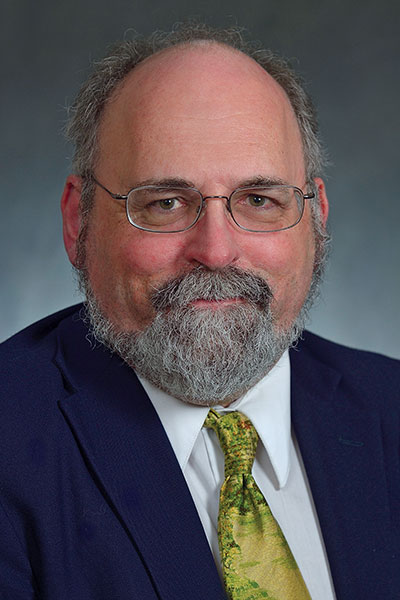
We have asked ourselves where we are when it comes to the application of AI technology in the field of lung cancer diagnosis and treatment. We’ve only scratched the surface in terms of knowledge and understanding of AI, it seems. Hence, it is imperative we learn more and determine how AI may influence and mold different fields that deal with thoracic malignancy: radiologic diagnostics, pathology, lung cancer risk assessment, drug discovery, robotic surgery, and radiation oncology. Within fields such as radiation oncology, we also must consider segmentation (contouring) of tumors, organs-at-risk for radiotherapy planning, and detection of in-field recurrences.
The fundamental question exists: How will AI enhance our understanding of thoracic malignancy and our treatment paradigms?
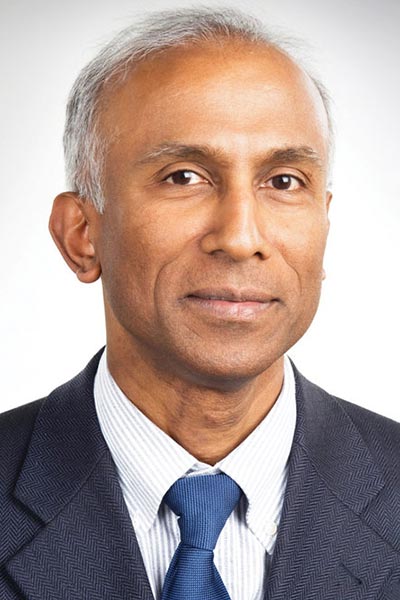
As an example, thoracic surgeons are already benefiting from numerous AI applications in robotic surgery. The use of robot-assisted thoracic surgery (RATS) is skyrocketing. According to Premier Healthcare Database, in the US in 2020, 43% of lobectomies were performed by RATS compared to 30% and 24% performed by VATS and open thoracotomies, respectively. Robotic surgery employs data science and AI by taking in information and providing analytics to surgeons to improve current and future procedures.
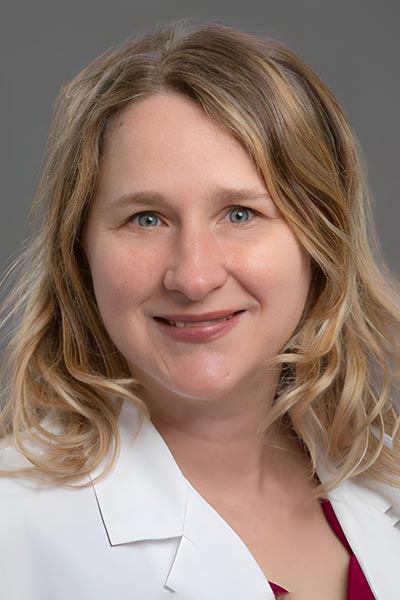
Radiation oncologists are also using AI in the context of stereotactic body radiation therapy (SBRT). In the context of real-time tracking of SBRT delivery, AI solutions and robotics can be used to precisely treat cancerous tumors providing personalized treatment to patients.
In the coming months, ILCN will explore these topics through a series of articles with comments from expert scientists on these specific aspects of lung cancer. Our series will begin with report that summarizes lung cancer fields where AI applications have been developed or are developing, such as radiology, histopathology, molecular biology, radiotherapy, medical oncology, and lung cancer risk prediction.
Like it or not, AI will be an intrinsic component of the diagnostic and therapeutic landscape; it is incumbent on us to understand its implications, limitations, and benefits so we can harness this technology rather than let it intrude upon our daily working lives.