DeepGEM, an artificial intelligence (AI) based whole slide imaging approach to cancer driver gene mutation screening, could provide accurate, affordable, and timely gene mutation predictions to guide targeted lung cancer treatment.
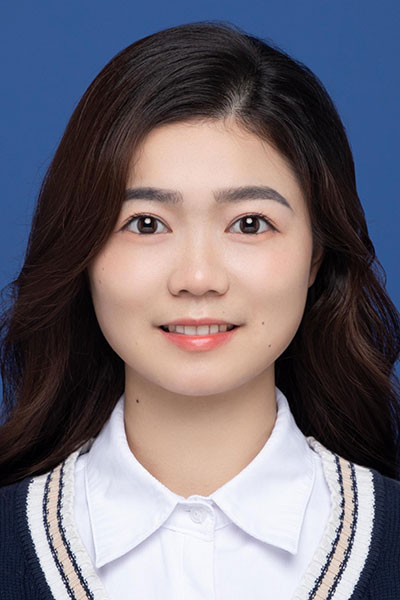
During a presentation at the session Predicting the Future: Novel Pathology Assessments and Imaging Biomarkers at the 2024 World Conference on Lung Cancer, experts introduced the audience to DeepGEM and its potential to become a new assistive technology for guiding lung cancer treatment.
DeepGEM can produce gene mutation maps that show the spatial distribution of mutations in each sample. Imaging is based on routinely acquired histology slides and can correlate genotype and phenotype to accurately predict the likelihood of clinically relevant oncogenic driver mutations in each tissue sample.
A validation trial using a public dataset called The Cancer Genome Atlas (TCGA) showed a median area under the curve (AUC) of 0.874 across diverse racial and ethnic samples compared to next-generation sequencing (NGS) testing.
“Rapid and precise detection of driver gene mutations is crucial for precision treatment,” said Shan Xiong, MD, doctoral candidate at China State Key Laboratory of Respiratory Disease & National Clinical Research Center for Respiratory Disease, First Affiliated Hospital of Guangzhou Medical University, Guangzhou, China.
For patients with lung cancer, NGS is the guideline-recommended method. However, it requires high-quality tissue samples and is time—and resource—consuming. As a result, NGS is not available for most patients, especially those in underdeveloped regions.
Earlier studies have found that EGFR, KRAS, ALK, ROS1, TP53, and LRP1B oncogenic driver mutations have specific histologic features that can be identified on slide imaging of both excisional and aspiration biopsy samples.
An internal data set of 1,717 whole slide images showed an AUC of 0.939 for excisional biopsies, 0.83 for aspirational biopsies, and 0.881 for the entire data set compared to NGS testing, Dr. Xiong reported. She said the results are a significant improvement compared to prior AI-based driver gene prediction efforts.
Validation on an external data set of 1,179 sides from 15 centers across China showed similar results with an AUC of 0.860 for excisional biopsies, 0.853 for aspiration biopsies, and 0.842 for the entire data set. Correlation ranged from 0.908 for EGFR mutations to 0.799 for LRP1B mutations.
Validation using 535 whole slide images from TCGA produced a median AUC of 0.872, ranging from 0.945 for EGFR to 0.858 for LRP1B. Comparison with a lymph node data set of 331 images had an AUC of 0.911 for EFGR and 0.882 for KRAS.
When compared to EGFR mutations confirmed by gene sequencing, patients with a high EGFR mutation probability, as predicted by DeepGEM, had significantly better survival outcomes than patients with a low predicted EGFR mutation probability (p = 0.024).
Spatial gene mutation maps generated by DeepGEM showing high-probability EGFR mutation regions were consistent with findings based on immunohistochemistry results.
“DeepGEM achieved robust and superior predictive performance across cancer genes of diverse types and racial backgrounds,” Dr. Xiong said. “The rapid prediction capabilities of DeepGEM allow for quicker decision-making in treatments, enabling patients with severe symptoms to receive targeted therapies sooner. DeepGEM presents opportunities for multi-gene detection and precision treatment in economically underdeveloped areas where genomic testing is unaffordable.”