Imagine lung cancer screening that begins with a single scan for everyone once they reach a certain age. And then, based on what is seen on that scan, a patient receives a personalized recommendation for a follow-up scan 1, 3, 5, or even 10 years later. The process requires no attempts to estimate tobacco or pollution or other exposures. Everything needed to predict if a patient is at high risk of developing lung cancer can be seen on a digital image analyzed by an artificial intelligence- powered algorithm.
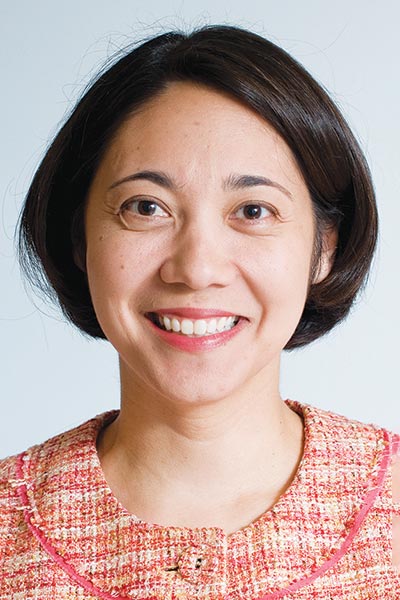
Many hope such a future is possible, including researcher Lecia V. Sequist, MD, MPH, who is Program Director of the Cancer Early Detection and Diagnostics Clinic and Landry Family Professor of Medicine at Harvard Medical School, Boston. Dr. Sequist is a member of the team that developed Sybil, an AI tool that can predict an individual’s risk for developing lung cancer up to 6 years into the future.1 Dr. Sequist recently spoke with ILCN about how AI may affect lung cancer screening and risk prediction.
ILCN: From a practical point of view, where are we in implementing AI in lung cancer screening?
Dr. Sequist: As you know, AI is a broad term. There are some instances where AI is already part of lung cancer screening. Many CT machines use AI. It is already baked into the machines. Some health systems are already using AI for quality control and the technical processing of scans.
But with the interpretation of the scans—and I think there is a lot of potential for AI in many different steps in that process—there are several companies that are developing proprietary AI algorithms to identify abnormalities, like pulmonary nodules, in a scan. When a patient has multiple scans over time, using AI can really help us better understand the risk that one of these nodules might be cancer. There are different programs that may help radiologists make sure that potential cancers are not missed.
The thing I’m excited about, and where my research focuses, is how we can use AI in another way. I’m interested in using AI to interpret images and predict might be at risk for cancer in the future, which is different than using it to determine what is happening in their body right now.
ILCN: Speaking of using AI for risk prediction, can it help us identify at-risk individuals who might otherwise slip through the cracks? For example, those without tobacco use history who, nevertheless, should still be screened?
Dr. Sequist: I am really hopeful about this possibility. One of the big problems with lung cancer is we don’t have a good understanding of why one person gets it, but another person does not. In the past, we have had a much too simplistic view of this, which was smoking as the one and only risk factor. Certainly, tobacco use is associated with lung cancer, but we don’t really understand why one person who smokes gets lung cancer and another person who smokes equally does not get lung cancer. And as you know, there is a startling rise in people getting lung cancer who either never smoked or who smoked decades and decades ago. I think it is a very complex issue.
One thing that is nice about AI is that you do not necessarily have to be able to quantify all the risks. As we learn that genetics can increase risk for lung cancer, as we learn that air pollution and other environmental exposures can increase risk for lung cancer, we don’t necessarily have to measure each one of those things. How could we? If we think estimating tobacco use is hard, try to estimate pollution exposure. It is an impossible task.
With AI, we can look at the images. We can see how someone’s lungs and entire chest appear. Even if we don’t understand how to measure an individual’s air pollution exposure, we can look at the result of the underlying genetics and biology of the person—how their lifetime of accumulated exposures biologically affected their lungs—to look for patterns. These patterns are probably too subtle or too complicated for a human radiologist to pick up, but that is where AI can be helpful. Pattern recognition is something that computers do really, really well.
AI can look at the anatomy and tell us if a person is at risk for developing cancer or tell us, conversely, that a person does not have the features it knows are associated with lung cancer development. This allows us to screen the people who are at the highest risk and follow them closely. It allows us to divorce our screening goals from the stigmatizing questions about tobacco use, which I think have really crippled us to date.
I’m really hopeful that this is going to be a whole new chapter in how we think about screening everybody for lung cancer—focusing on those who are highest risk.
ILCN: That is exciting. With AI, can a single low-dose CT scan or chest X-ray, without the presence of a nodule, be a predictor of lung cancer?
Dr. Sequist: We are currently doing research to try and understand that, but from the data we have so far, it does seem like one CT scan is sufficient to predict risk at least out to 6 years. It may not be sufficient for predicting risk for decades, but certainly over the short- or medium-term, one chest CT may be enough. It is an interesting question about whether this type of information could come from an x-ray. I don’t know. There are certainly researchers that have looked at predicting lung cancer from x-rays, and that is certainly cheaper and easier for patients to go through. However, the level of detail of the anatomy is much coarser with an x-ray. I think it remains to be seen yet, whether an x-ray could function in the same way.
Getting to a point where we can predict risk with a single scan will allow us to screen in a way that is similar to how we conduct other screenings. For example, colon cancer screening. With current guidelines, everyone is supposed to get a baseline colonoscopy at age 45. Then depending on what is seen on that colonoscopy, the patient may not need another one for 10 years. If there are lots of polyps, the patient may need another one in 5 years or 3 years. It is risk-adjusted and personalized based on what is seen the first time.
The AI tool that we developed, or something like it, could help us create the same kind of paradigm in lung cancer screening—everyone gets screened at a certain age and then, depending on the risk stratification, a patient may get annual scans or may not need another one for 10 years.
ILCN: As the role of AI in screening expands, to what extent will radiologists come on board? Do they perceive AI as a threat to their autonomy when it comes to film interpretation?
Dr. Sequist: Radiologists are a key component of this research. I cannot speak for all radiologists, but the radiologists I’ve worked with are extremely welcoming of this technology. The tools that I’m developing are not intended to take the place of a radiologist. They are doing something that is parallel to what the radiologist is doing. The point of the AI tools is to try to help improve the radiologist’s skill. It is not taking away from the radiologists, in my opinion. Rather, it enhances their ability to do their job well and to do it more efficiently so that they can spend less time in front of the computer screen and more time interacting with patients or doing their research.
ILCN: Do you think AI will enable us to detect the “BRCA1” of NSCLC? Or is this a pipedream?
Dr. Sequist: BRCA1 was such a paradigm-changing discovery. It allows us to predict who is at the highest risk of several cancers. Medical care for a BRCA1 carrier is starkly different compared to the care for those who do not carry BRCA1. I don’t think we will find an equivalent gene like that in lung cancer, but that doesn’t mean that the same paradigm shift won’t happen. It may just be more complicated to figure out who is at the highest risk. It may not necessarily be a genetic mutation that makes them high risk. It may be a compilation of several factors. So yes, I do think that Sybil1 and similar tools that look at personalized risk could help us get to that type of paradigm.
ILCN: Finally, can AI facilitate increased lung cancer screening throughout the world? How?
Dr. Sequist: The majority of countries in the world don’t have screening programs, so we have nowhere to go but up. I do think AI, in general, can help bring improved healthcare to low- and middle-income countries, rural places, marginalized populations, etc.
Lung cancer screening Is complicated in some settings in the world where CT scans just don’t exist for hundreds of miles. AI can help to some extent, especially if programs are based off things that are readily available to those patients. You asked me earlier whether we could do this with the chest x-ray. That would be prime example of something that would be much easier to facilitate. We know that a radiologist looking at a chest x-ray is not a good way to screen for lung cancer, but maybe AI developed for that purpose could perform better than a human.
References
- 1. Mikhael PG, Wohlwend J, Yala A, et al. Sybil: A Validated Deep Learning Model to Predict Future Lung Cancer Risk From a Single Low-Dose Chest Computed Tomography. J Clin Oncol. 2023;41(12):2191-2200. doi:10.1200/JCO.22.01345