Assessment of tumor material by the pathology laboratory has become a cornerstone of treatment of virtually all thoracic oncology patients. This applies not only to initial diagnosis and prognosis in early-stage disease, but also to advanced stage scenarios, where the prediction of response to a rapidly increasing list of systemic therapy options depends on results from a correspondingly expansive toolbox of immunohistochemical and molecular tests.1
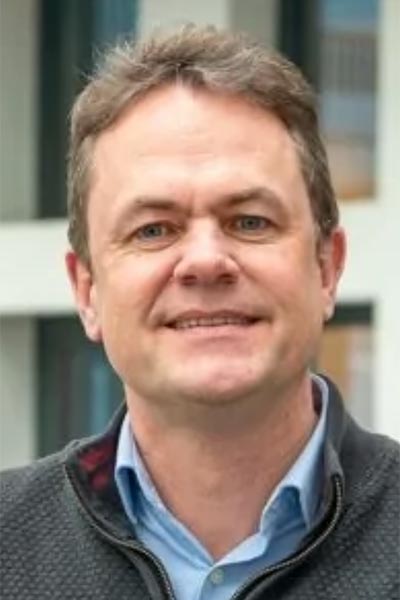
The drive for increasingly tailored treatment in turn increases the demands on diagnostic thoracic pathologists, who must maintain in-depth knowledge of rapidly changing tumor classification and staging systems as well as of treatment options and their accompanying predictive markers. Even for subspecialty expert thoracic pathologists, the inherent ambiguities and complexity of current classification and scoring methodologies limit diagnostic accuracy and reproducibility, as has been borne out by many interobserver reproducibility studies.2,3,4
Conversely, current classifications and tests are hampered by an incomplete knowledge of more detailed (morphological) tumor subclassification that could be more predictive of prognosis and treatment response than current standards. These limitations could potentially be addressed and overcome by the application of artificial intelligence (AI) in thoracic pathology.
Digital Pathology
Use of AI in routine pathology first depends upon transition to digital pathology reporting.5,6,7,8 Increasingly, diagnostic labs are scanning and digitizing glass slides, with the computer screen gradually replacing the microscope as a pathologist’s main tool. The motivation for this is primarily improved efficiency and ease of image use, however the great value of digital images as a base for digital analysis is being increasingly recognized. Digital microscopic images permit easy, time- and space-independent access, which allows for more efficient workflow and easier quality control (images do not deteriorate and are not lost); access to images by multiple people simultaneously; and easy and fast consultation with colleagues worldwide without the need for time-consuming physical transport of slides. It also enables integration of images with other disciplines such as radiology.
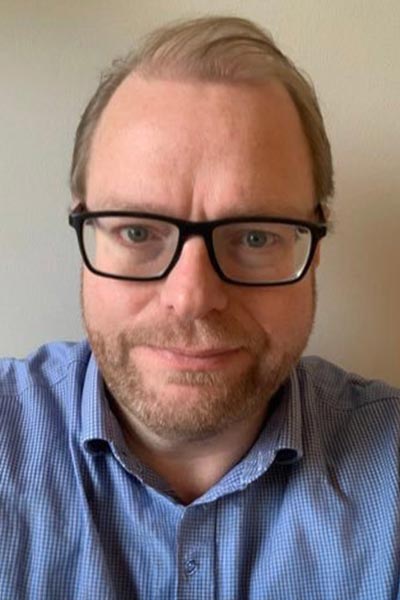
Image Segmentation and Quantification
Some of the more time-consuming and error-prone tasks of pathologists in thoracic oncology involve the identification of specific cellular subsets based on their appearance in routine histochemical stains and immunohistochemistry. Examples are counting mitoses for classification of bronchopulmonary neuroendocrine neoplasms or the scoring of tumor cell expression of PD-L1 in cases where anti-PD-(L)1 check point inhibition is considered. AI algorithms can perform these tasks more quickly, more consistently, and potentially more accurately than human operators.9,10
For instance, deciding whether a tumor exceeds a cut-off of 50% PD-L1 tumor cell expression to qualify a patient for treatment with mono-immunotherapy is a difficult problem if the overall level is near the 50% mark, especially if heterogeneous staining is seen. Moreover, we now know that psychological attributes may influence the scoring of individual pathologists.11 AI, on the other hand, can rapidly assess thousands of cells for PD-L1 expression, arriving at an unbiased and precise estimation of overall expression levels.
Morphological Classification
The World Health Organization classification of thoracic tumors is the basis for diagnosis of virtually all thoracic malignancies.12 While this is a tremendously helpful resource in ensuring consistency of pathology practice across the globe, it is an imperfect classification due to the inherent ambiguity that pathologists encounter in the delineation of specific entities in real life. Thus, morphological characteristics are paramount when deciding whether to classify a high-grade neuroendocrine carcinoma as small cell or large cell, and this often results in serious treatment implications. We also know that interobserver concordance is suboptimal in making this distinction.3,13
Similarly, adenocarcinoma classification has for many years been known to hold significant prognostic value, but its full introduction into clinical practice has been lagging, in part due to perceived difficulties around reproducibility.2 Algorithms that aid morphological classification could assist even very experienced thoracic pathologists, and considerable progress has been made in this field in recent years.14
Genomic Classification
The detection of targetable genomic alterations by DNA and RNA sequencing has become a mainstay for prediction of response to various forms of systemic therapy, especially tyrosine kinase inhibitors.1 While the techniques currently employed in testing for these characteristics are highly sensitive and specific, they are not universally available or fundable. It could therefore be interesting to explore possibilities for HE-based alteration prediction, focusing on mutations, fusions, and amplification of known driver genes. If applicable in routine practice, this could alter the care pathway to the upfront use of fast, affordable, and universally available HE stains for either selecting or excluding cases for more sophisticated and expensive molecular diagnostic tests. As the reliability and accuracy of such algorithms increases, molecular testing could perhaps be replaced by HE-based prediction of specific alterations, at least for a subset of patients.15
Prognostication and Response Prediction
AI is also likely to hold potential for prognosis and prediction independent of current classification systems, known genomic alterations, or expression patterns of tumor cells or the surrounding microenvironment. In designing such models, histopathological images are used for training, validation and testing without the need for annotations other than clinical outcome.16 This could offer opportunities for prognostication in early-stage disease, thus potentially defining subgroups which would benefit from (neo)adjuvant treatment and more extensive local therapy, while withholding such potentially harmful and financially toxic treatment from those who are not likely to derive any survival benefit from it. Similarly, such approaches may provide more accurate response prediction in patients who qualify for (neo-)adjuvant or palliative systemic therapy than current predictive markers.
Unsupervised Classification and ‘Explainable’ AI
The final, and potentially most rewarding rung on the AI ladder, when it comes to exploring novel morphological features and their related biological entities and clinical significance, is the concept of unsupervised classification in conjunction with so-called ‘explainable’ AI. In this methodology, unsupervised clustering is performed on components (such as smaller tiles) of non-annotated images, and these clusters can then be used to explore their prognostic or predictive relevance in correlation with clinical outcome data.17 Once validated, these clusters and the resulting overall classification of the entire tumor could then be used in isolation for prognostic and predictive purposes. However, perhaps most excitingly, this could also provide a vantage point for unbiased exploratory analysis through the concept of ‘explainable AI.’ This means that individual segments, tiles, or cells that hold the most relevant outcome information can be investigated for specific morphologic features that could serve to refine current classifications and to be translated to routine pathology practice, and/or these areas of the tumor could be subjected to orthogonal techniques such as mutation profiling or spatial transcriptomics for more in-depth exploratory molecular analysis.
While we are still in the early phases of the application of AI in thoracic pathology, this field holds great promise for better definition of prognosis and prediction of therapy response for our patients. It has the potential to facilitate affordable worldwide access to such techniques and will ultimately promote equity of outcomes for the global patient community. It is also likely to serve as a flywheel for rapid expansion of our knowledge of the biology of thoracic malignancies. These significant benefits have been recognized by the IASLC Pathology Committee, which has recently founded an AI working group to coordinate, lead, and support such initiatives within the association and beyond.
References
- 1 Smeltzer MP, Wynes MW, Lantuejoul S, et al. The International Association for the Study of Lung Cancer Global Survey on Molecular Testing in Lung Cancer. J Thorac Oncol. 2020;15(9):1434-1448. doi:10.1016/j.jtho.2020.05.002.
- 2 Warth A, Muley T, Meister M, et al. The novel histologic International Association for the Study of Lung Cancer/American Thoracic Society/European Respiratory Society classification system of lung adenocarcinoma is a stage-independent predictor of survival. J Clin Oncol. 2012;30(13):1438-1446. doi:10.1200/JCO.2011.37.2185.
- 3 Thunnissen E, Borczuk AC, Flieder DB, et al. The Use of Immunohistochemistry Improves the Diagnosis of Small Cell Lung Cancer and Its Differential Diagnosis. An International Reproducibility Study in a Demanding Set of Cases. J Thorac Oncol. 2017;12(2):334-346. doi:10.1016/j.jtho.2016.12.004.
- 4 Dacic S, Travis W, Redman M, et al. International Association for the Study of Lung Cancer Study of Reproducibility in Assessment of Pathologic Response in Resected Lung Cancers After Neoadjuvant Therapy. J Thorac Oncol. 2023;18(10):1290-1302. doi:10.1016/j.jtho.2023.07.017.
- 5 Niazi MKK, Parwani AV, Gurcan MN. Digital pathology and artificial intelligence. Lancet Oncol. 2019;20(5):e253-e261. doi:10.1016/S1470-2045(19)30154-8.
- 6 Bera K, Schalper KA, Rimm DL, Velcheti V, Madabhushi A. Artificial intelligence in digital pathology – new tools for diagnosis and precision oncology. Nat Rev Clin Oncol. 2019;16(11):703-715. doi:10.1038/s41571-019-0252-y.
- 7 Hanna MG, Ardon O, Reuter VE, et al. Integrating digital pathology into clinical practice [published correction appears in Mod Pathol. 2021 Oct 13;:] [published correction appears in Mod Pathol. 2021 Nov 9;:]. Mod Pathol. 2022;35(2):152-164. doi:10.1038/s41379-021-00929-0.
- 8 Baxi V, Edwards R, Montalto M, Saha S. Digital pathology and artificial intelligence in translational medicine and clinical practice. Mod Pathol. 2022;35(1):23-32. doi:10.1038/s41379-021-00919-2.
- 9 Aubreville M, Wilm F, Stathonikos N, et al. A comprehensive multi-domain dataset for mitotic figure detection. Sci Data. 2023;10(1):484. Published 2023 Jul 25. doi:10.1038/s41597-023-02327-4.
- 10 Hondelink LM, Hüyük M, Postmus PE, et al. Development and validation of a supervised deep learning algorithm for automated whole-slide programmed death-ligand 1 tumour proportion score assessment in non-small cell lung cancer. Histopathology. 2022;80(4):635-647. doi:10.1111/his.14571.
- 11 Butter R, Hondelink LM, van Elswijk L, et al. The impact of a pathologist’s personality on the interobserver variability and diagnostic accuracy of predictive PD-L1 immunohistochemistry in lung cancer. Lung Cancer. 2022;166:143-149. doi:10.1016/j.lungcan.2022.03.002.
- 12 Nicholson AG, Tsao MS, Beasley MB, et al. The 2021 WHO Classification of Lung Tumors: Impact of Advances Since 2015. J Thorac Oncol. 2022;17(3):362-387. doi:10.1016/j.jtho.2021.11.003.
- 13 den Bakker MA, Willemsen S, Grünberg K, et al. Small cell carcinoma of the lung and large cell neuroendocrine carcinoma interobserver variability. Histopathology. 2010;56(3):356-363. doi:10.1111/j.1365-2559.2010.03486.x.
- 14 Lami K, Ota N, Yamaoka S, et al. Standardized Classification of Lung Adenocarcinoma Subtypes and Improvement of Grading Assessment Through Deep Learning. Am J Pathol. 2023;193(12):2066-2079. doi:10.1016/j.ajpath.2023.07.002.
- 15 Coudray N, Ocampo PS, Sakellaropoulos T, et al. Classification and mutation prediction from non-small cell lung cancer histopathology images using deep learning. Nat Med. 2018;24(10):1559-1567. doi:10.1038/s41591-018-0177-5.
- 16 Akram F, Wolf JL, Trandafir TE, Dingemans AC, Stubbs AP, von der Thüsen JH. Artificial intelligence-based recurrence prediction outperforms classical histopathological methods in pulmonary adenocarcinoma biopsies. Lung Cancer. 2023;186:107413. doi:10.1016/j.lungcan.2023.107413.
- 17 Pan X, AbdulJabbar K, Coelho-Lima J, et al. The artificial intelligence-based model ANORAK improves histopathological grading of lung adenocarcinoma. Nat Cancer. 2024;5(2):347-363. doi:10.1038/s43018-023-00694-w.